Autonomous AI systems, also known as agentic AI, are reshaping industries by making independent decisions without constant human intervention. From managing investment portfolios to powering driverless vehicles, they bring efficiency and innovation to tasks once limited by human constraints. However, the independence of these systems raises ethical concerns, especially when decisions lead to significant societal outcomes, as seen in AI’s role in determining job applications or credit approvals.
Global discussions about responsible AI have intensified following incidents such as Tesla’s autonomous vehicle crashes and the discovery of algorithmic bias in recruitment software. Regulatory bodies like the European Commission have taken action through initiatives like the AI Act, aiming to address these challenges through accountability, transparency, and risk-based classifications.
These discussions are essential for businesses navigating legal obligations and societal expectations. Ethical frameworks ensure that AI development goes beyond technological optimisation, focusing instead on long-term societal benefits. Companies that implement these practices will likely gain public trust and reduce regulatory risks.
Accountability in autonomous systems
As AI systems make decisions independently, clarifying who takes responsibility when things go wrong is essential. The complexity of autonomous decision-making blurs traditional lines of accountability. A self-driving car that causes an accident raises the question: should the manufacturer, software developer, or system operator be held liable? The 2018 fatal Uber self-driving car crash is a case in point, as legal disputes arose over whether the human safety driver or the company should bear responsibility.
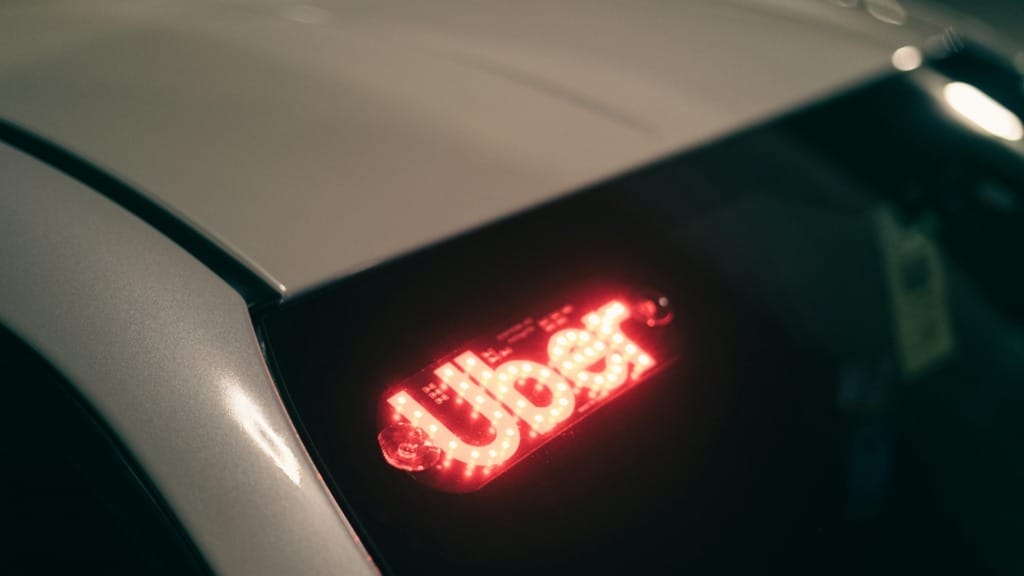
Legal systems worldwide are struggling to define liability for AI-related incidents. The EU’s AI Act attempts to resolve this by placing responsibility on developers and users of high-risk systems. In the US, state-level regulations such as California’s autonomous vehicle laws outline how companies must assume liability during trials and deployments. Such measures are designed to prevent companies from avoiding responsibility when failures occur.
Internal accountability frameworks within organisations are equally important. Companies like Microsoft and Google have established AI ethics boards that review product development, ensuring that risks are considered at every stage. By involving legal and ethical experts early, these companies minimise the chances of deploying AI systems without safeguards.
Accountability doesn’t involve legal compliance; it reflects the organisation’s commitment to preventing harm. Developers can implement technical fail-safes, such as human-in-the-loop designs, where critical decisions require human verification. By addressing accountability holistically, organisations can enhance the safety and reliability of AI applications.
Transparency in AI decision-making
While accountability focuses on determining responsibility after an AI failure, transparency ensures that decision-making processes can be scrutinised and understood before things go wrong. Many AI systems, especially those based on machine learning, function as “black boxes,” where even developers may not fully understand how certain outputs are generated. This opacity can undermine public trust, especially when decisions affect access to resources or opportunities.
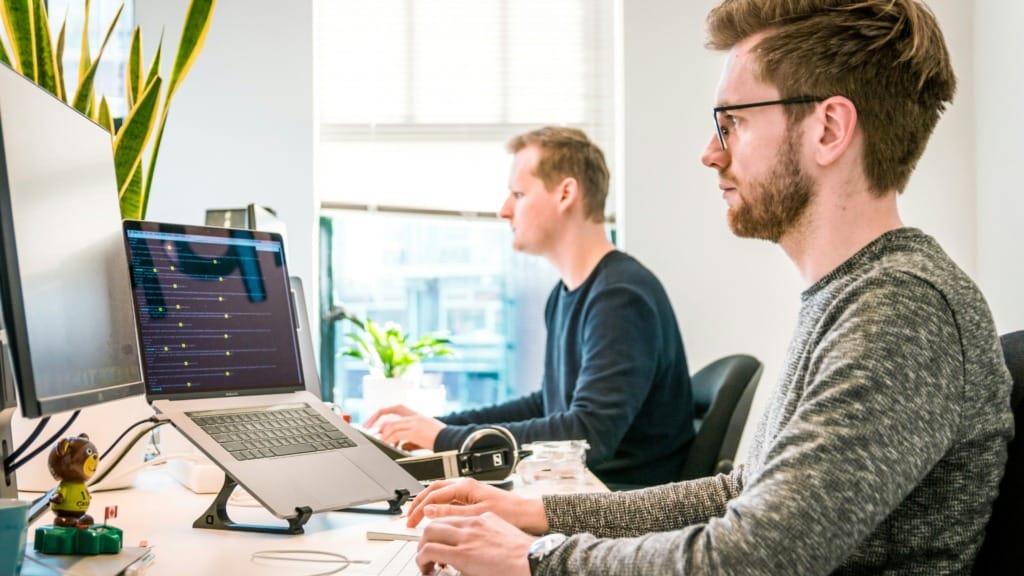
Explainable AI (XAI) is central to achieving transparency. Unlike traditional opaque models, XAI provides interpretable outcomes by revealing the factors influencing a decision. IBM’s AI FactSheets provide detailed documentation on model design, testing, and performance, making it easier for regulators and stakeholders to assess potential risks. This ensures AI decisions are not just accepted passively but actively understood.
Balancing transparency with proprietary concerns is challenging. Companies may hesitate to disclose AI processes out of fear that competitors could reverse-engineer their models. To address this, tiered transparency approaches have emerged, where detailed technical information is disclosed selectively to regulators while simplified explanations are provided to the public.
Governments are stepping in to ensure that transparency is upheld. The EU’s AI Act mandates that users must be informed when interacting with AI and given explanations for decisions that significantly affect them. Such regulations protect individual rights and encourage responsible AI design, as developers are incentivised to build systems that can withstand scrutiny.
Addressing bias in autonomous agents
While transparency focuses on making AI decisions understandable, bias addresses whether those decisions are fair and equitable. Bias in AI can arise from training data that reflects historical inequalities or algorithmic processes that unintentionally favour particular groups. For instance, Amazon’s AI recruitment tool demonstrated gender bias because it was trained on data from a male-dominated hiring environment.
Addressing bias requires a combination of data hygiene, algorithm design, and continuous monitoring. Developers can start by auditing training datasets to ensure they are representative and free of discriminatory patterns. Google’s Fairness Indicators tool helps developers test for disparities across different demographic groups, allowing biases to be detected before deployment.
Algorithmic audits are another key step. Organisations should regularly evaluate their models using fairness metrics to assess whether outcomes differ across subgroups. Companies like Facebook have begun conducting external audits to detect unintended discriminatory impacts within their algorithms. These independent evaluations improve accountability and ensure that internal teams aren’t overlooking biases.
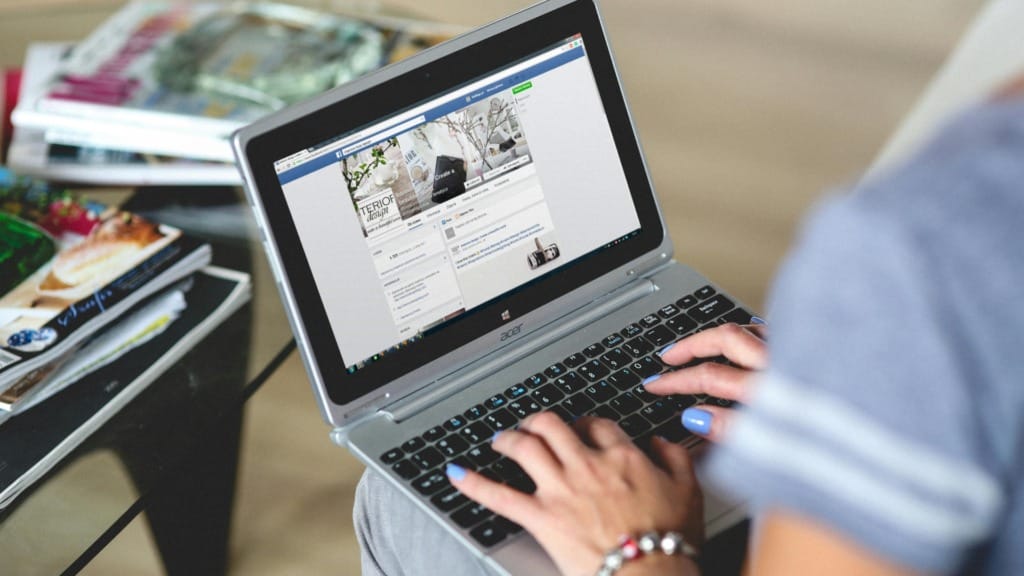
Addressing bias is about building systems that reflect societal values. When AI systems produce biased outcomes, they risk undermining trust and creating legal liabilities. Integrating bias mitigation measures into the development lifecycle ensures that AI systems serve all users equitably, fostering long-term trust in technology.
Frameworks for responsible AI development
Bias mitigation is one aspect of responsible AI development, but ensuring long-term responsibility requires comprehensive frameworks. Ethical guidelines like the OECD’s AI Principles offer a starting point, promoting values like fairness, transparency, and human-centred design. However, implementing these principles effectively requires governance structures tailored to each organisation.
Internal governance models ensure that ethical concerns are not treated as an afterthought. For example, Google’s AI ethics board conducts regular reviews of projects and identifies risks that may arise from data usage or deployment strategies. These organisations reduce the risk of unforeseen harm by embedding ethics within the development process.
Cross-disciplinary collaboration is another essential component. AI development involves more than just engineers—input from ethicists, sociologists, and legal experts is necessary to understand societal risks. For instance, Microsoft has collaborated with human rights groups to evaluate how its AI products affect vulnerable communities. These partnerships provide a more holistic view of potential risks and solutions.
Feedback mechanisms ensure that AI systems remain aligned with ethical standards even after deployment. Regular user feedback and post-deployment audits help organisations identify issues as they arise. By continuously refining their AI systems, companies can ensure that their technologies remain responsive to societal needs and evolving norms.
Aligning AI behaviour with human values
Responsible frameworks guide development, but aligning AI with human values ensures that the outcomes of its decisions benefit society. Unlike static rules, values are dynamic and vary across cultures, making value alignment a complex challenge. Automated decision-making systems that ignore societal norms can lead to public backlash, as seen when AI systems deny financial assistance based on income without considering broader human contexts.
Mechanisms like reinforcement learning with human feedback (RLHF) enable developers to teach AI systems how to prioritise desirable outcomes. OpenAI has used this technique to train models like ChatGPT, ensuring that responses align with user expectations and ethical considerations. By incorporating human guidance, AI systems are better able to reflect complex social expectations.
Cultural differences in values require tailored solutions. Privacy norms in Europe, for example, differ significantly from those in the United States, necessitating regional adaptations in AI deployments. Companies can ensure broader acceptance and avoid legal disputes by designing AI systems to comply with local regulations.
Value alignment must be an ongoing process. As societal values evolve, AI systems should be regularly updated to reflect these changes. Continuous dialogue with policymakers, academics, and the public ensures that AI remains aligned with contemporary ethical standards and delivers positive societal outcomes.